Main Theater[East hall 1]
Feb. 2, 2023 (Thu.)
【nano tech Special Symposium】Data-driven nanotechnology - from materials to devices and systems
【Organizer】nano tech executive committee
【Time】9:30-11:30
Pre-Registration required
Full
A new frontier of materials development based on multicrystalline informatics
9:35-10:10 Pre-Registration required
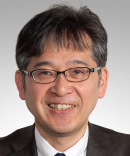
Nagoya University
Graduate School of Engineering
Professor
Prof. Noritaka Usami
【Profile】
1993 Completed Master's program, Graduate School of Engineering, The University of Tokyo
1994 Research Associate, Research Center for Advanced Science and Technology, The University of Tokyo
1998 Doctor of Engineering, The University of Tokyo
2000 Associate Professor, Institute for Materials Research, Tohoku University
2013-present: Professor, Graduate School of Engineering, Nagoya University
2018-2020 Senior Science and Technology Policy Fellow, Cabinet Office, Government of Japan
2017-present: Professor, Center of Integrated Research for Future Electronics, Institute for Materials and Systems for Sustainability, Nagoya University
2022-present: Professor, Center for the Creation of a Decarbonized Society, Institute of Innovation for Future Society, Nagoya University
Specialized in Crystal Engineering and Applied Physics, Crystal Materials Informatics
【Abstract】
We pioneer the development of multicrystalline materials informatics by linking experimental, theoretical, computational, and data sciences to construct a new materials development method that answers the fundamental question, "What kind of crystal should we make and how? In this presentation, I will introduce the research basis we have established for silicon, including 3D visualization of multicrystalline structure and dislocation cluster distribution, prediction of crystal orientation distribution, network analysis describing the multicrystalline structure, feature extraction of dislocation cluster generation points, and quantification of carrier recombination rate at grain boundaries. The presentation will also include the integration of these research bases to deepen materials science, efficient search for manufacturing processes for high-performance materials, and application of these bases to research and development of other materials.
Automatic Design/Synthesis of Analog Integrated Circuits using Machine Learning
10:10-10:45 Pre-Registration required
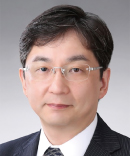
Kyoto Institute of Technology
Professor
Prof. Nobukazu Takai
【Profile】
Prof. Nobukazu Takai received the B.E. and M.E. degrees from Tokyo University of Science, Tokyo , Japan, in 1993, and 1995, respectively and Ph.D. degree from Tokyo Institute of Technology, Tokyo, Japan, in 1999. After working as an assistant professor at Tokyo Polytechnic University and associate professor at Gunma University, he is now a Professor at Kyoto Institute of Technology. He engaged in the research of DC-DC Converter, analog filters, and analog integrated circuit design and now his main interest lies in the field of automatic design/synthesis design of analog integrated circuit using machine learning. Prof. Takai is a member of the Institute of Electrical Engineers of Japan, the Institute of Electronics, Information and Communication Engineers, and the Institute of Electrical and Electronics Engineers.
【Abstract】
This presentation introduces methods applying machine learning in the analog integrated circuit design flow. I will introduce the results of applying machine learning to the circuit topology selection that satisfy spec., which was conventionally performed by competent designers using their experience and knowledge. Also, I will report the automatic synthesis of the circuit topology using a computer. By applying deep learning to the automatic synthesis, I will show that a new circuit, which was not included in the training data, is synthesized.
Energy efficient information processing by physical reservoir computing
10:45-11:20 Pre-Registration required
IBM Research - Tokyo
Research Staff Member
Dr. Toshiyuki Yamane
【Abstract】
Reservoir computing is a variant of recurrent neural networks with three layers; input, randomly fixed and training-free reservoir for high dimensional nonlinear transformation of input, and readout with linear signal processing.
Thanks to this architectural simplicity, reservoir computing is attracting much attention as a cost-effective machine learning algorithm.
Furthermore, physical reservoir computing is actively investigated where reservoir layer is replaced by physical dynamics to achieve more power efficiency and higher speed than software implementation.
Among various types of physics, optics and spintronics implementations of reservoirs are most extensively studied.
In this talk, we review the recent progress of optical and spintronic reservoir computing and discuss future industrial applications such as edge computing.